Revolutionizing Transportation: Understanding Training Data for Self Driving Cars
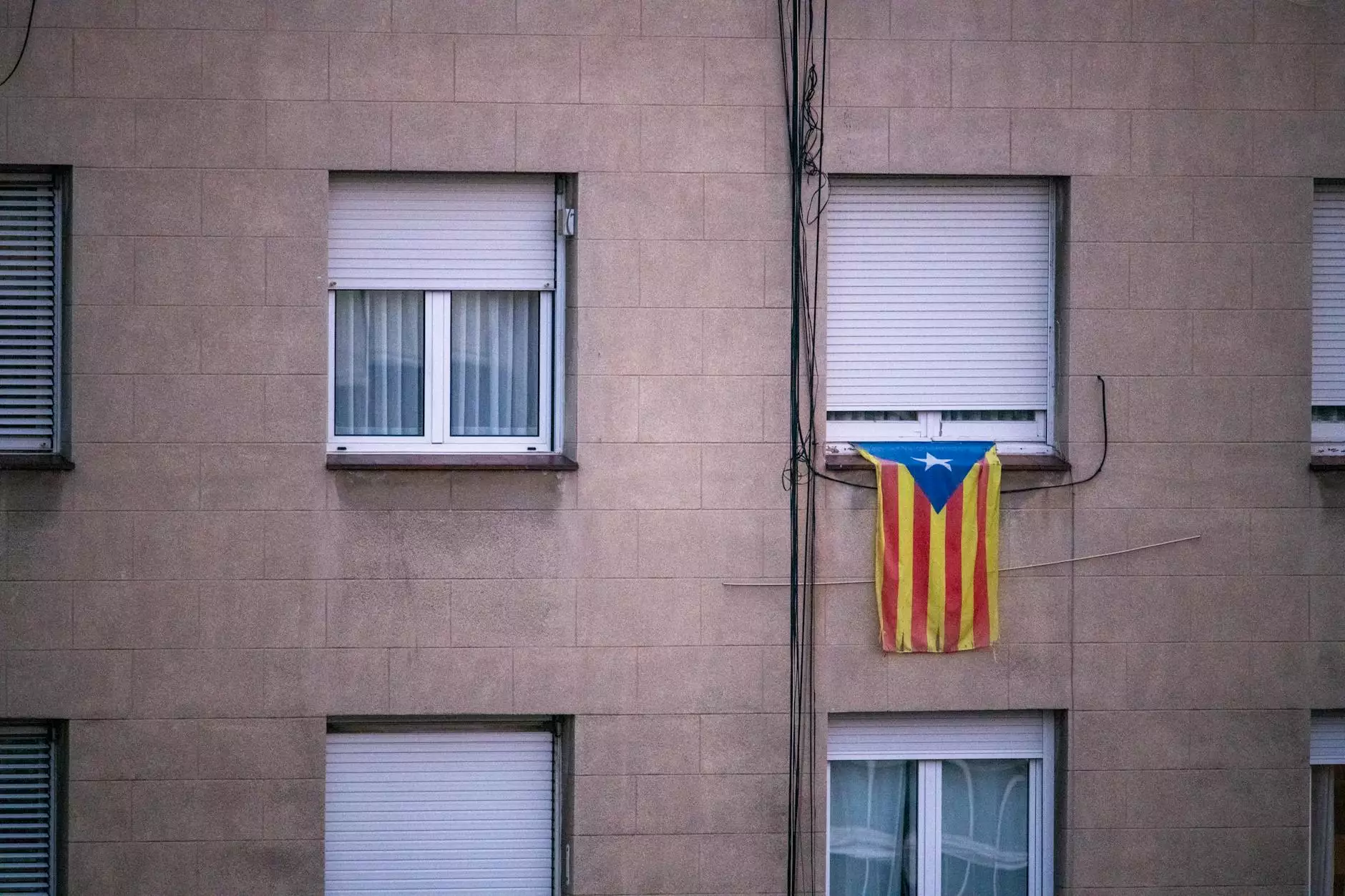
The Significance of Training Data in Autonomous Vehicles
The emergence of self-driving cars has revolutionized the way we think about transportation. At the core of this technological advancement is training data for self driving cars, which is essential for developing systems that can operate vehicles autonomously. In this article, we will delve deeply into the importance of this training data and explore how it shapes the future of transportation.
What is Training Data?
Training data refers to the extensive datasets used to train machine learning algorithms. For self-driving cars, this data includes various scenarios captured through sensors such as cameras, LIDAR, and radar. The algorithms analyze this data to learn how to perceive their environment, make decisions, and navigate safely.
- Sensor Data: Information from cameras, LIDAR, and radar providing a 360-degree view of the surroundings.
- Vehicle Dynamics: Data on how the car reacts in different situations, such as acceleration, braking, and steering.
- Environmental Context: Weather conditions, road conditions, and traffic regulations that impact driving behavior.
The Role of Quality Data in Training Models
The quality of training data directly influences the performance of autonomous systems. High-quality data ensures that machine learning models can effectively generalize, improving their reliability on the road. Data must be:
- Comprehensive: Covering a wide range of scenarios, from urban driving to highway conditions.
- Diverse: Including variations in weather, lighting, and potential obstacles.
- Accurate: Free from errors that could lead to incorrect interpretations by the AI.
Sources of Training Data
Self-driving vehicle technology relies on a collection of diverse data sources. These sources can be categorized as follows:
1. Real-World Driving Data
In-field data collected from real-world driving experiences offers invaluable insights. Companies deploy fleets of vehicles equipped with sensors that record driving behaviors, performance, and environmental interactions.
2. Simulation Data
Simulations play a critical role in generating training data without the safety risks associated with real-world driving. Engineers create virtual environments to simulate complex driving scenarios, enhancing the training dataset.
3. Public Dataset Collections
Numerous research institutions and organizations publish public datasets containing labeled images and driving scenarios. These resources are crucial for benchmarking algorithms and facilitating collaboration in the industry.
The Benefits of Self-Driving Cars for Businesses
The implementation of autonomous vehicle technology offers a plethora of benefits for various industries:
- Increased Efficiency: Self-driving cars can optimize routes and reduce travel time, leading to faster delivery services.
- Cost Reduction: By minimizing the need for human drivers, businesses can significantly cut labor costs.
- Enhanced Safety: Autonomous vehicles have the potential to reduce accidents caused by human error, improving overall road safety.
Challenges in Collecting Training Data
Despite its importance, collecting quality training data for self driving cars poses several challenges:
- Regulatory Concerns: Regulations on data privacy and safety can hinder the collection of valuable data.
- Data Annotation: Labeling vast amounts of data is labor-intensive and requires significant resources.
- Environmental Variability: Training data must account for differences in geography, weather, and other conditions, requiring extensive datasets.
Future Directions in Training Data Development
The future of self-driving cars hinges on innovative approaches to training data. Some promising directions include:
1. Synthetic Data Generation
Innovations in generating synthetic data using advanced simulation technology can help expand datasets. This data mimics real-world conditions closely, aiding in training robust models.
2. Continuous Learning
Implementing systems that allow vehicles to continue learning from real-world interactions enables constant refinement of models, enhancing overall performance.
3. Collaborative Data Sharing
Creating open data networks among industry players fosters collaboration and accelerates the development of autonomous vehicle technology.
Conclusion: Embracing the Future of Transportation
The journey toward fully autonomous vehicles is heavily reliant on the availability and quality of training data for self driving cars. As companies in the home services, keys, and locksmiths industries, it is essential to stay informed about these advancements and consider how technology can be integrated into business operations to improve efficiency, safety, and customer satisfaction.
Keymakr: Pioneering Innovation in Home Services
At Keymakr, we understand the transformative power of technology in enhancing our services. By embracing innovations such as autonomous vehicles and expanding our knowledge of training data for self driving cars, we strive to remain at the forefront of the industry.