Harnessing Malware Detection Using Machine Learning for Enhanced Cybersecurity
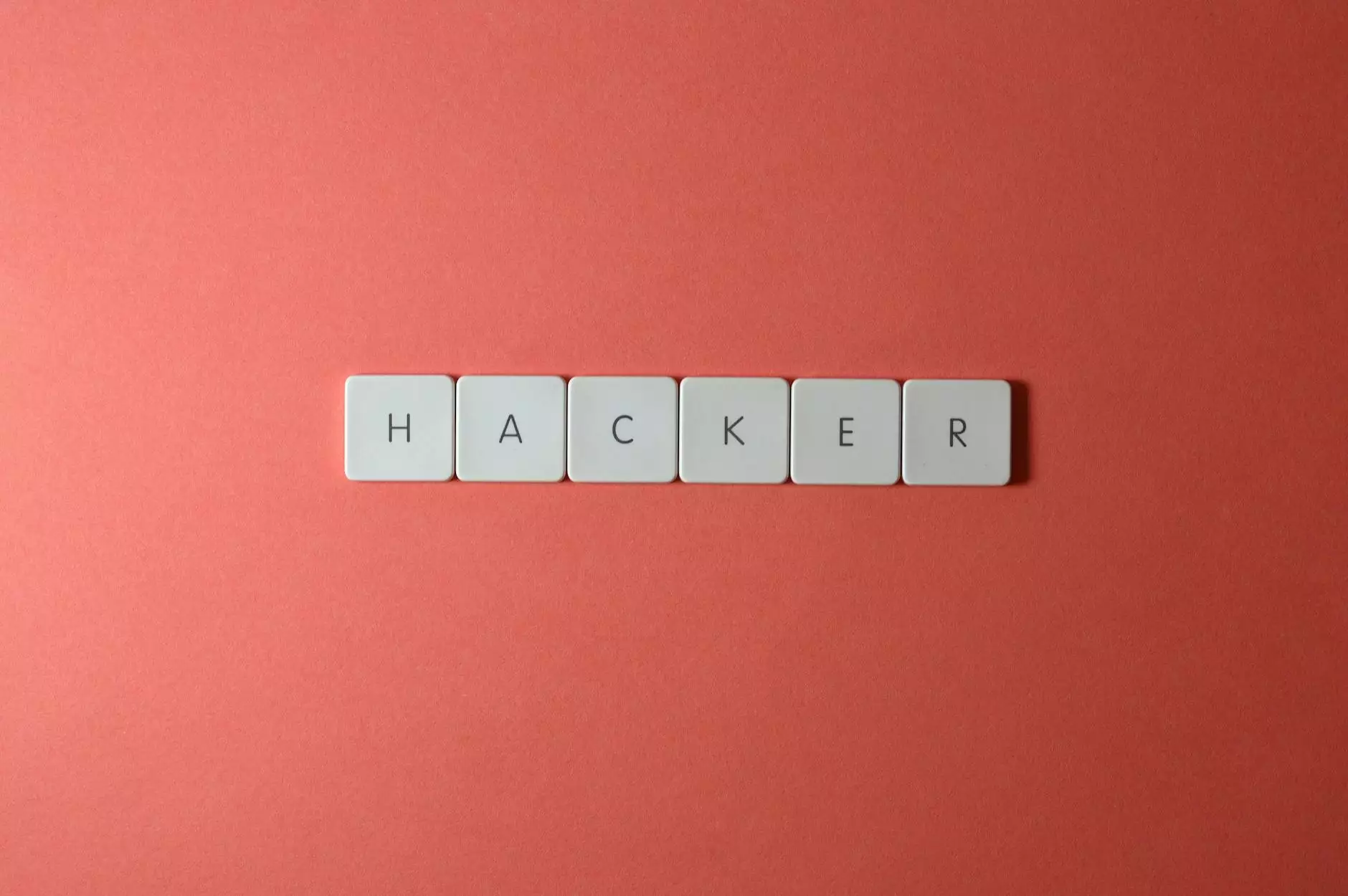
In today's rapidly evolving technological landscape, cybersecurity has become a paramount concern for businesses and individuals alike. Malware—malicious software—poses a significant threat, leading to data breaches, financial loss, and reputational damage. As malicious actors become increasingly sophisticated, traditional methods of malware detection are proving inadequate. This is where malware detection using machine learning revolutionizes cybersecurity, offering businesses a powerful tool to combat these threats effectively. Here, we explore the profound impact of machine learning on malware detection and how companies like Spambrella are at the forefront of this technological revolution.
Understanding Malware and Its Threat Landscape
To appreciate the importance of malware detection using machine learning, it's essential to understand what malware is and the various forms it can take. Malware encompasses a range of malicious software types, including:
- Viruses: Malicious code that attaches itself to clean files and spreads throughout a computer system.
- Worms: Standalone software that replicates itself to spread to other computers.
- Trojans: Disguised as legitimate software, but capable of harming a system.
- Ransomware: Malware that locks or encrypts files, demanding a ransom for access.
- Spyware: Software designed to gather information about a person or organization without their knowledge.
As these threats evolve, the need for advanced detection methods becomes increasingly critical. Traditional signature-based detection methods are no longer sufficient, as they rely on known malware signatures and cannot detect previously unseen threats.
The Role of Machine Learning in Cybersecurity
Machine Learning (ML) refers to the subset of artificial intelligence that involves algorithms that improve automatically through experience. In the realm of cybersecurity, machine learning enhances malware detection by analyzing vast amounts of data to identify patterns, anomalies, and potential threats. The advantages of using machine learning for malware detection include:
1. Enhanced Detection Rates
Machine learning models can process enormous datasets—far beyond human capability. By learning from historical data, these models can identify emerging threats and new variants of malware with impressive accuracy. This leads to improved detection rates compared to traditional methods.
2. Real-Time Threat Assessment
One of the critical benefits of deploying machine learning in malware detection is the ability to perform real-time analysis. Businesses can identify and respond to threats as they occur, minimizing potential damage and improving overall cyber resilience.
3. Reduction in False Positives
False positives can be detrimental, leading to unnecessary downtime and operational disruptions. Machine learning algorithms continuously improve their accuracy, helping to reduce false positive rates and allowing security teams to focus on genuine threats.
4. Predictive Capabilities
Machine learning can identify trends and predict potential future attacks by analyzing data over time. This predictive capability helps organizations stay one step ahead of cybercriminals, implementing preventative measures before breaches occur.
How Malware Detection Using Machine Learning Works
The process of malware detection using machine learning involves several key steps:
Data Collection
The first step is collecting and aggregating large datasets, which include:
- Network traffic logs
- File attributes and metadata
- Behavioral patterns from user interactions
- Known malware signatures for training
Data Preprocessing
The collected data must be preprocessed to ensure quality and relevance. This involves cleaning the data, removing redundancies, and transforming it into a suitable format for machine learning algorithms.
Feature Extraction
Feature extraction is crucial in identifying the characteristics that will allow the model to differentiate between benign and malicious software. For example, attributes like file size, execution time, and system calls can serve as key indicators in the decision-making process.
Training the Model
Using labeled datasets (both benign and malicious), machine learning models are trained to recognize patterns and make predictions. Different algorithms such as decision trees, support vector machines, and deep learning networks can be tested to determine which yields the best performance.
Testing and Validation
After training, the model is tested with unseen data to assess its accuracy and reliability. Validation ensures that the model performs well in real-world conditions, minimizing the chances of false negatives and positives.
Deployment and Continuous Learning
Once validated, the model is deployed in a real-world environment where it continuously analyzes incoming data. The system is designed to learn and adapt over time, improving its accuracy and efficacy as it encounters new malware variants.
Challenges in Implementing Machine Learning for Malware Detection
Despite its advantages, integrating machine learning in malware detection comes with challenges:
1. Data Privacy Concerns
Collecting data for training purposes raises data privacy issues. Organizations must ensure they comply with regulations such as GDPR to protect user data.
2. Complexity of Model Training
Training machine learning models requires significant expertise, resources, and time. Organizations may struggle to find the right talent to develop and maintain these systems.
3. Evolving Threats
The dynamic nature of malware means that algorithms need constant updates and retraining, requiring ongoing investment and resources.
Case Studies: Successful Implementation of Machine Learning in Malware Detection
Numerous organizations have successfully implemented malware detection using machine learning to enhance their cybersecurity posture. Some notable case studies include:
1. Spambrella's Approach
Spambrella, a leader in IT services and security systems, has embraced machine learning for malware detection across its network security solutions. By integrating advanced machine learning algorithms, Spambrella has significantly reduced malware attack rates, thereby protecting its clients from evolving threats.
2. IBM's Watson for Cyber Security
IBM has deployed its Watson AI to offer advanced threat intelligence solutions. Watson's machine learning capabilities allow it to analyze billions of events and develop insights that alert security teams about potential threats, reducing response time and improving incident management.
3. Cisco's Security Intelligence
Cisco's security solutions employ machine learning to identify and mitigate threats across a range of environments. Their systems assess user behavior and network traffic to pinpoint anomalies indicative of malware, ensuring rapid response to cyber threats.
The Future of Malware Detection Using Machine Learning
The future of malware detection using machine learning is promising as advancements in artificial intelligence continue to evolve. Organizations will benefit from:
1. Improved Algorithms
As research in artificial intelligence progresses, we can expect more sophisticated algorithms capable of detecting even the most elusive malware strains.
2. Enhanced Collaboration
Collaboration between businesses and cybersecurity organizations will pave the way for shared intelligence, significantly improving collective defense against malware.
3. Integration with Other Technologies
Combining machine learning with other technologies like blockchain, threat intelligence platforms, and cloud computing will enhance overall cybersecurity strategies.
Conclusion
In a world where cyber threats are omnipresent, malware detection using machine learning emerges as a crucial component of modern cybersecurity strategies. With the ability to detect, predict, and respond to threats in real-time, machine learning provides businesses with a robust defense against an ever-evolving threat landscape. Organizations like Spambrella exemplify how leveraging these technologies can lead to safer digital environments, underscoring the necessity for continuous innovation in cybersecurity practices.
As we move forward, investing in machine learning capabilities is not just beneficial; it's essential for any organization looking to safeguard its assets, reputation, and customer trust in the digital age.